Introduction
Hodgkin lymphoma (HL), first described by Thomas Hodgkin, is a lymphoma that represents about 1% of all de novo neoplasms occurring every year worldwide [1]. According to the World Health Organization classification, HL comprises 2 types of HL, classical Hodgkin lymphoma (accounting for 95% of all HL cases) and nodular lymphocyte predominant HL (accounting for 5% of all HL cases) [2, 3]. The incidence of HL was found to be increased in teenagers and patients older than 55 years [4]. However, the mechanisms underlying HL initiation and progression remained unclear.
Long non-coding RNAs (lncRNAs), a kind of ncRNAs over 200 bps in length, have been found to play key roles in cancer progression by regulating gene expression through transcriptional and post-transcriptional mechanisms [5–7]. For example, lncRNA EPIC1 promoted breast tumorigenesis by interacting with MYC to induce cell-cycle-related gene expression [8]. MALAT1 interacted with the PRC2 complex to regulate inflammatory gene expression in diabetes [9]. However, the roles of lncRNAs in HL remained unknown. Until now, only two reports have indicated that lncRNAs were involved in HL progression. Tayari et al. [10] identified HL-specific lncRNAs by using microarray profiling. Leucci et al. [11] also found that MALAT1 could bind to miR-9 to regulate its targets expression in the cHL cell line L-428. Exploring the potential functions of lncRNAs in HL could provide useful information to identify novel biomarkers for HL.
In this study, we focused on identifying relapse-related lncRNAs in HL by analyzing the Gene Expression Omnibus (GEO) dataset GSE17920. Next, we conducted co-expression network analysis and bioinformatics analysis for relapse-related lncRNAs in HL. Moreover, we constructed a relapse-related lncRNA-miRNA-mRNA network to explore the potential mechanisms of these lncRNAs. We trust that the work will provide potential new therapeutic and prognostic targets for HL.
Material and methods
LncRNA classification pipeline
In this work, a pipeline was utilized to identify lncRNA expression pattern in HL, which was described by Zhang et al. [12].
Microarray data and data preprocessing
In the work, we analyzed GSE17920 to identify relapse-related mRNAs and lncRNAs in Hodgkin lymphoma by downloading GEO datasets. A total of 9 early relapse HL and 24 late relapse HL samples were included in GSE17920, which was reported by Christian et al. All samples were obtained from Hodgkin’s lymphoma patients.
Functional group analysis
Here, we performed Gene Ontology (GO) analysis and Kyoto Encyclopedia of Genes and Genomes (KEGG) analysis to predict the potential roles of genes by using the DAVID system (https://david.ncifcrf.gov/).
Results
Identification of relapse-related mRNAs and lncRNAs in Hodgkin lymphoma
In the present study, we analyzed the publicly available gene expression dataset GSE17920 to identify relapse-related mRNAs and lncRNAs in Hodgkin lymphoma. A total of 9 early relapse HL and 24 late relapse HL samples were included in GSE17920. We firstly analyzed differentially expressed mRNAs in the GSE17920 database and found that 130 genes were up-regulated and 1209 genes were down-regulated in late relapse HL compared with early relapse HL (Figure 1 A).
Figure 1
Identification of relapse-related mRNAs and lncRNAs in Hodgkin lymphoma. A – Hierarchical clustering analysis showed differential mRNAs and lncRNA expression in the HL by using GSE17920. B – GSE17920 analysis showed that 18 lncRNAs were dysregulated in late relapse HL compared with late relapse HL
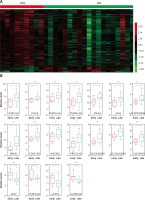
We compared the lncRNA expression patterns between early relapse HL and late relapse HL. A total of 18 lncRNAs were dysregulated in late relapse HL compared with early relapse HL (Figure 1 A). Among them, GGTA1P, PCBP1-AS1, GK3P and IL10RB-AS1 were down-regulated and PGM5-AS1, HCG18, CHRM3-AS2, PSMD6-AS2, SNHG6, LOC102606465, LOC100190986, GAS5, MIR29B2, PRKCQ-AS1, ITGB2-AS1, MIR142, LOC101060091 and LINC00926 were up-regulated in late relapse HL samples (Figure 1 B).
Construction of a relapse-related lncRNA-mRNA co-expression network
Furthermore, we constructed a relapse-related lncRNA-mRNA co-expression network by calculating the Pearson correlation coefficient of the lncRNA-mRNA pair. In this study, only the lncRNA-mRNA pair with |R| > 0.65 was selected for network construction. Our results revealed that the relapse-related lncRNA-mRNA co-expression network consisted of 18 lncRNAs and 762 DEGs (Figure 2). HCG18, PGM5-AS1, PSMD6-AS2, and SNHG6 acted as key lncRNAs in this network by affecting more than 200 mRNAs.
GO and KEGG analysis of differentially expressed lncRNAs
To obtain the potential functions of differentially expressed lncRNAs, we used GO and pathway analysis. The results of GO analysis showed that the upregulated genes were mainly involved in regulating responses to organic substances, immune system processes, responses to stress, cellular responses to organic substances, regulation of immune system processes, responses to chemicals, responses to stimuli, cellular responses to chemical stimuli, regulation of responses to stimuli, cell surface receptor signaling pathways, and cell activation (Figure 3 A). Meanwhile, we found that the molecular functions of upregulated genes were mainly involved in protein binding, enzyme binding, carbohydrate derivative binding, ion binding, glycoprotein binding, identical protein binding, monosaccharide binding, antigen binding and small molecule binding (Figure 3 B).
Figure 3
GO and KEGG analysis of differentially expressed lncRNAs in HL. (A–B) GO analysis shows differentially expressed lncRNA-related biological process analysis (A) and molecular function analysis (B). C – KEGG pathway analysis reveals differentially expressed lncRNA-related KEGG pathway analysis. D – Hub-network 1 contained 53 nodes and 458 edges. E – Hub-network 2 contained 37 nodes and 188 edges. F – Hub-network 3 contained 39 nodes and 135 edges
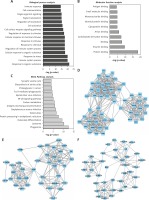
The results of KEGG pathway analysis demonstrated that upregulated lncRNAs were mainly associated with the phagosome, lysosome, osteoclast differentiation, protein processing in endoplasmic reticulum, tuberculosis, Staphylococcus aureus infection, antigen processing and presentation, and carbon metabolism (Figure 3 C).
Construction of relapse-related lncRNA-mediated PPI networks
We next constructed relapse-related lncRNA-mediated PPI networks based on the information in the STRING database. Furthermore, the Mcode plugin was further used to identify key modules (cut-off ≥ 3 and the nodes with edges ≥ 3-core). In total, 17 hub-networks were identified in this study. The top 3 hub-networks with more than 20 nodes are shown in Figure 4. Hub-network 1 contained 53 nodes and 458 edges (Figure 3 D), hub-network 2 contained 37 nodes and 188 edges (Figure 3 E), and hub-network 3 had 39 nodes and 135 edges (Figure 3 F).
Construction of relapse-related lncRNA-mediated ceRNA networks
We also constructed relapse-related lncRNA-mediated ceRNA to study the potential mechanism of lncRNAs regulating mRNA expression. We selected positively correlated lncRNA-mRNA pairs with |R| > 0.65 to build ceRNA networks. Then, we predicted the potential interactions of miRNA-lncRNA and miRNA-mRNA using StarBase. As shown in Figure 4, 6 lncRNAs (PCBP1-AS1, HCG18, GAS5, PSMD6-AS2, PRKCQ-AS1, SNHG6), 116 mRNAs and 121 miRNAs were included in this ceRNA network. Interestingly, we found that PCBP1-AS1 and HCG18 were the key regulators in this network. According to our analysis, we found that HCG18 could sponge more than 75 miRNAs in the HL relapse-related progression and PCBP1-AS1 could regulate more than 60 mRNAs by competitive binding to hsa-miR-34a-5p, hsa-miR-9-5p, hsa-miR-34c-5p, hsa-miR-539-5p, hsa-miR-122-5p, hsa-miR-124-3p, hsa-miR-449a, hsa-miR-449b-5p, hsa-miR-491-5p, and hsa-miR-506-3p.
Discussion
Hodgkin lymphoma (HL) is the main common lymphoma throughout the western countries [16]. Relapse of Hodgkin lymphoma after treatment is a major therapeutic challenge. However, the molecular mechanisms involved in the relapse of HL remained unclear. Emerging studies have shown that lncRNAs play key roles in cancer progression. In HL, only two reports have indicated that lncRNAs were involved in HL progression. For example, Tayari et al. identified HL-specific lncRNAs by using microarray profiling [10]. In this study, we analyzed relapse-related lncRNAs in HL using the public dataset GSE17920. A total of 18 lncRNAs were dysregulated in the relapse progression of HL. Among them, GGTA1P, PCBP1-AS1, GK3P and IL10RB-AS1 were down-regulated and PGM5-AS1, HCG18, CHRM3-AS2, PSMD6-AS2, SNHG6, LOC102606465, LOC100190986, GAS5, MIR29B2, PRKCQ-AS1, ITGB2-AS1, MIR142, LOC101060091 and LINC00926 were up-regulated in late-relapse HL samples compare to early-relapse HL. To the best of our knowledge, this is the first study to reveal relapse-related lncRNAs in HL.
Among these lncRNAs, SNHG6 and GAS5 have been reported to be key regulators in cancers. SNHG6 could promote esophageal squamous cell carcinoma, glioma, gastric cancer and hepatocellular carcinoma cell proliferation though ceRNA mechanisms [17–20]. GAS5 acted as a tumor suppressor involved in various cancers, including breast cancer, lung cancer, liver cancer and gastric cancer [21–24]. However, the molecular roles of these lncRNAs in HL remained unknown. In the present study, we first constructed relapse-related lncRNA-mRNA co-expression networks which included 18 lncRNAs and 762 mRNAs. Moreover, we performed bioinformatics analysis. Our results showed these lncRNAs were mainly involved in regulating responses to organic substances, immune system processes, responses to stress, cellular responses to organic substances, regulation of immune system processes, and phagosome and lysosome-related pathways. Finally, we constructed relapse-related lncRNAs mediated PPI networks. These results strongly suggested that lncRNAs also play important roles in relapse progression of HL.
The competitive endogenous RNA (ceRNA) hypothesis showed that lncRNAs could competitively bind to miRNAs to regulate protein-coding gene expression [25]. Emerging studies have shown that ceRNA play crucial roles in the development and progression of human diseases, such as prostate cancer, gliomas, liver cancer, and diabetes [26]. The ceRNA hypothesis provides an important mechanism to explain how lncRNAs regulate disease progression. Here, we constructed relapse-related lncRNA-mediated ceRNA to explore the potential mechanism of lncRNAs regulating mRNA expression. A total of 6 lncRNAs (PCBP1-AS1, HCG18, GAS5, PSMD6-AS2, PRKCQ-AS1, SNHG6), 116 mRNAs and 121 miRNAs were included in this ceRNA network. Interestingly, we found that PCBP1-AS1 and HCG18 were the key regulators in this network. According to our analysis, we found that HCG18 could sponge more than 75 miRNAs and PCBP1-AS1 could regulate more than 60 mRNAs. PCBP1-AS1 was a novel lncRNA involved in oral squamous cell carcinoma progression [27] and lncRNA HCG18 promoted intervertebral disc degeneration by sponging miR-146a-5p and regulating TRAF6 expression [28].
In conclusion, we identified a total of 18 lncRNAs that were found to be dysregulated between early relapse and late relapse HL. Furthermore, we constructed co-expression networks for these relapse-related lncRNAs. Bioinformatics analysis revealed that these lncRNAs were significantly associated with immune system processes, responses to stress, and responses to chemicals. Moreover, we constructed a relapse-related lncRNA-miRNA-mRNA network to explore the potential mechanism of these lncRNAs. OF note, HCG18 and PCBP1-AS1 were identified as key lncRNAs in HL relapse. Although the results must be confirmed in a prospective clinical trial on a larger group of patients, we trust that this work will give a potential new therapeutic and prognostic target for HL.