Diabetic nephropathy (DN) is a major complication of diabetes, with high morbidity and mortality, and it is a common cause of end-stage renal failure [1]. The recommended therapies for DN include hyperglycaemia control, inhibition of the renin-angiotensin-aldosterone system, and blood pressure control [2, 3]. Meanwhile, reducing proteinuria by therapy may also improve renal outcomes in DN patients [4]. However, although angiotensin-converting enzyme inhibitors (ACEIs) have been shown to protect renal function, their effect on delaying DN progression remains inadequate. Recently, traditional Chinese medicine (TCM) has been widely used to treat diabetes and its complications, including DN [5]. Among these, tangshen formula (TSF) has been reported to alleviate kidney injuries in patients with type 2 diabetes [6]. TSF is composed of seven different herbs: Astragali Radix (Chinese name: Huangqi), Ramulus euonymi (Guijianyu), Rehmanniae radix (Dihuang), Aurantii Fructus (Zhiqiao), Corni Fructus (Shanzhuyu), Rhei Radix et Rhizoma (Dahuang), and Notoginseng Radix et Rhizoma (Sanqi). However, the pharmacological mechanism of action of TSF in the treatment of DN is still unclear because of its multi-component and multi-target characteristics. Network pharmacology is closely connected with the application of multiple omics and systems biology techniques, which provides the possibility to explore the compounds and relevant targets of TCM [7]. Thus, this study aimed to investigate the pharmacological mechanisms of TSF in DN using a network pharmacology approach.
Gene expression data of GSE90842 was downloaded from the Gene Expression Omnibus database (http://www.ncbi.nlm.nih.gov/geo) based on the GPL16570 platform, including nine samples from db/m mice (NC group), db/db mice (DN group), and DN mice administered TSF for 12 weeks (TSF group), with three samples in each group. After data standardization, the differentially expressed genes (DEGs) in the DN vs. NC and TSF vs. DN groups were screened using the limma package. P < 0.05 and |log fold change| > 0.263 were set as the thresholds. VENN analysis was applied to screen the DN-related DEGs that were reversed after TSF treatment. These genes were used in subsequent analyses.
TSF was composed of seven different herbs, and their compounds were searched using the Traditional Chinese Medicine Integrated Database (TCMID, http://183.129.215.33/tcmid/search/). Target genes of these compounds were predicted using the Bioinformatics Analysis Tool for Molecular mechANisms (BATMAN) database (http://bionet.ncpsb.org/batman-tcm/), and targets with a score cut-off > 20 were selected. These targets were subsequently integrated with the identified DEGs to obtain common genes for further analyses.
Pathway enrichment analysis of these genes was performed using the R package clusterProfiler; p < 0.05 and gene count ≥ 2 were considered to be statistically significant. Based on the herb-chemical-DEG-pathway data generated by the above step, Cytoscape software (version 3.7.1) was employed to construct the pharmacological network.
A total of 1784 and 1468 DEGs were identified in the DN vs. NC and TSF vs. DN groups, respectively. Next, 249 (92 upregulated and 157 downregulated) DEGs related to both DN and TSF treatments were selected for analysis. The number of chemical constituents or targets of each herbal medicine ingredient were identified (Table I). Meanwhile, 23 common genes were identified by integrating these targets and DEGs. Pathway enrichment analysis showed that genes were involved in four Kyoto Encyclopaedia of Genes and Genomes (KEGG) pathways, including neuroactive ligand-receptor interaction, the cAMP signalling pathway, synaptic vesicle cycle, and serotonergic synapse.
Table I
The number of chemical constituents or targets for each herbal
Based on the above results, the pharmacological network was structured to identify the associations between the herbs, components, targets, and pathways. As shown in Figure 1, the network was composed of 80 nodes (6 herbs, 47 components, 23 targets, and 4 pathways) and 704 edges. Several active compounds of TSF with higher degrees were observed in this pharmacological network such as progesterone, tritriacontanol, and hesperidin, indicating that they might be multi-target and multi-effect compounds for the treatment of DN. In addition, we observed that genes such as SLC6A4, ABCG1, and NR1I2 might be hub proteins in the pharmacological network.
Figure 1
The pharmacological network of TSF. The purple triangle indicates herbal medicine, the yellow quadrangle indicates the compound, the red dot indicates upregulated targets, the green diamond indicates downregulated targets, and the blue hexagon indicates the KEGG pathway
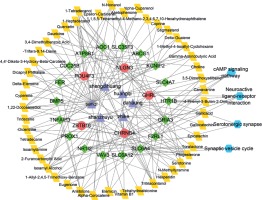
In the present study, a network pharmacology approach was utilized to reveal the potential pharmacological mechanisms of TSF in DN. Several key compounds and targets have attracted the attention of researchers. Progesterone is a female sex hormone that plays a role in DN pathology. Al-Trad et al. [8] observed that progesterone treatment could substantially reduce the urinary albumin to creatinine ratio, which could decrease the early manifestations of DN. Moreover, hesperidin is a flavanone glycoside with antioxidant and antihyperglycaemic effects [9]. Previous evidence indicates that hesperidin plays a vital role in protecting the kidney in DN induced by high fat levels [10]. Taken together, we speculated that progesterone and hesperidin, important chemical compounds in TSF, might play a role in the prevention and treatment of DN.
Pharmacological network analysis showed that SLC6A4, NR1I2, and ABCG1 might be responsible for the TSF against DN. SLC6A4 has been reported to play a role in the development of diabetes. For example, Xiu et al. [11] suggested that genetic polymorphisms in SLC6A4 had a marked effect on patients with type 2 diabetes in southern China. In this study, pathway analysis showed that SLC6A4 was involved in the serotonergic synapse. Epidermal serotonergic synapses might have important clinical significance in sensory dysfunctions, such as the loss of tactile sensitivity in patients with diabetes or inflammatory diseases [12]. Inflammation played a crucial role in the progression of DN [13], and its anti-inflammatory effects could be successfully applied to clinical treatments in the next few years. Thus, SLC6A4 might play a role in DN treatment via affecting the serotonergic synapse pathway. Another gene, NR1I2 (also known as PXR), is considered a key gene in this network. A previous study indicated that PXR was upregulated in the diabetic kidney and might modulate the course of DN by activating pregnane X receptor-related target genes [14]. In this study, we found that the expression level of NR1I2 was downregulated after TSF treatment, suggesting that NR1I2 might serve as a potential therapeutic target for DN. Furthermore, proteins encoded by ABCG1 may regulate cellular lipid homeostasis and are involved in macrophage cholesterol and phospholipid transport. Abnormal lipid metabolism is closely associated with the pathogenesis of DN, and renal lipid metabolism-related genes, such as ABCG1, may serve as specific therapeutic targets to slow the progression of glomerulosclerosis [15]. Therefore, ABCG1 might act in the treatment of DN by affecting lipid homeostasis; however, further investigation is warranted to better understand the role of ABCG1 in DN.
The connections between the targets of chemical components in herbs and specific diseases could be revealed by a network pharmacology approach. However, the number of samples included in this analysis was small, which might have affected the accuracy of the results. In addition, the components and targets detected in the present study were based on bioinformatics analysis. Thus, further studies focusing on the animal models or clinical trials are required to validate our findings.
In conclusion, network pharmacological analysis provided a theoretical foundation for understanding the molecular mechanisms of TSF in DN treatment. The results demonstrated that several compounds, including progesterone and hesperidin, represented the key chemicals in the TSF. In addition, SLC6A4, NR1I2, and ABCG1 might be key factors regulated by TSF in the treatment of DN.