Introduction
Parapneumonic pleural effusion (PPE) is associated with a variety of infectious pulmonary diseases [1]. Currently, the prevalence of PPE is increasing in all age groups [2–4]. Approximately 40% of patients hospitalized with pneumonia have varying degrees of pleural effusion. Patients with PPE are more likely to have a longer duration of fever and a more serious condition than those with pneumonia alone. Moreover, such patients have a higher mortality and worse prognosis than those with only pneumonia [5]. Therefore, we should pay more attention to patients with PPE.
Since the completion of the Human Genome Project, the goal of research has shifted from genomic information to the study of proteins, which has led to a new research direction – proteomics. Proteomics is the study of the mechanisms of disease by analysing protein compositions, protein modification states, quantitative assays, differential expressions, qualitative identifications, and protein interactions, with the core techniques including isolation and identification of proteins, study of protein structure, and analysis and prediction of protein function. In the current study, we questioned whether there are differences in the energy metabolism and signal transduction in the pleural cavity in patients with PPE. Thus, we investigated the expressions of differential proteins in PPE by proteomic techniques and performed GO and KEGG analyses on the differential proteins.
Material and methods
Study subjects
In the present study, a total of 20 patients with PPE, who were hospitalized in the Department of Respiratory Medicine of Baoding First Central Hospital from February 2018 to August 2018, were included, comprising 10 patients with uncomplicated PPE (UPPE) and 10 patients with complicated PPE (CPPE). These patients were divided into Group A and Group B, respectively [5].
Inclusion criteria [6]
(1) New-onset cough, expectoration, and other respiratory symptoms or aggravation of an existing cough, expectoration, and purulent phlegm. (2) Fever (axillary temperature over 37.3°C). (3) Physical examination revealed signs of pulmonary consolidation with or without wet rales. (4) WBC > 10 × 109/l revealed by routine blood test. (5) Imaging examination: Chest CT showed flaky, patchy infiltrative opacities or interstitial changes together with pleural effusion (chest CT or thoracic ultrasonography). The included patients had to meet the above conditions, except for pulmonary vasculitis, pulmonary embolism, pulmonary insufficiency, non-infectious interstitial pulmonary disease, pulmonary oedema, pulmonary tumours, tuberculosis, and pulmonary eosinophilic infiltration.
Diagnostic criteria for UPPE and CPPE
Pleural fluid was collected and examined after each patient was admitted to the hospital. According to Light’s criteria, those with phases 1–2 were enrolled in the UPPE group, and those with phases 3–5 were enrolled in the CPPE group.
Light’s criteria: (1) Phase 1: Small amount of pleural effusion with an area of less than 1 cm on chest CT scan. (2) Phase 2: Chest CT scan of pleural effusion greater than 1 cm; pleural fluid with glucose > 2.24 mmol/l; pH > 7.2; LDH < 3 times the upper limit of the normal serum value; negative pleural fluid culture. (3) Phase 3: 7.00 < pH of the pleural effusion < 7.20; glucose in the pleural effusion > 2.24 mmol/l; LDH > 1000 U/l; negative pleural fluid culture. (4) Phase 4: Pleural effusion without coating and non-purulent in appearance; pH < 7.0, glucose in the pleural effusion < 2.24 mmol/l, or positive pleural fluid culture. (5) Phase 5: Multiple encapsulated effusions; pH < 7.0, glucose in the pleural effusion < 2.24 mmol/l, or positive pleural fluid culture. (6) Phase 6: Purulent pleural effusion with free or solitary package of the pleural fluid. (7) Phase 7: Multiple encapsulated purulent effusions, >5 mm increase in the thickness of the mural pleural.
Methods
According to Light’s criteria, the patients were divided into groups A and B, with the former as the UPPE group and the latter as the CPPE group. There were 10 cases in each group.
Sample collection
In all enrolled patients, pleural puncture or chest tube drainage was performed. A total of 10 ml of the pleural fluid from the first sample was retained and centrifuged at 3000 rpm for 10 min; the supernatant was separated. Then, 40 µl of specimen from each patient in each group was combined to form the samples for group A and group B. The samples were stored at –80°C in a refrigerator.
Protein extraction and quantitative quality control
The proteins were extracted using the ProteoExtract Albumin/IgG Abundance Extraction Kit by Merck. (1) Preparation of the sample solution: 60 µl of sample was mixed with 540 µl of ProteoExtract Albumin/IgG Binding Buffer. (2) The upper cover of the Albumin/IgG Extraction Column was removed, and with the upside-down, the column storage solution was discarded and the lower cover of the Albumin/IgG Extraction Column removed. (3) The column was balanced by 850 µl of the ProteoExtract Albumin/IgG Binding Buffer, with the flowing solution discarded. (4) The sample solution in step 1 was passed through the column connected in step 2, and the outflowing solution was collected. (5) The sample solution prepared in step 1 was placed in the Albumin/IgG Extraction Column, and the outflowing solution was collected. (6) Next, 600 µl of the ProteoExtract Albumin/IgG Binding Buffer was added to clean the column, and the outflowing solution was collected. (7) The procedures in the previous step were repeated, and all outflowing solution was collected. (8) Then, 8 ml of TCA/acetone (1 : 4) was added to the protein extraction solution, which was mixed well and kept at 4°C for 1.5 h. (9) At 4°C, the solution was centrifuged at 6000 rpm for 40 min. (10) Next, 400 µl of ice-cold acetone was added to each sample 4 times to break up the precipitate. The solution was kept at –40°C for 0.5 h, 4°C, and was centrifuged at 15,000 rpm for 15 min. The supernatant was discarded. (11) The procedures in steps 3–4 were repeated, and the solution was precipitated at –40°C for 0.5 h. (12) At 4°C, the solution was centrifuged at 15,000 rpm for 15 min and then dried for 15 min. (13) Finally, the solution was dissolved in 60 µl of 8 M urea and blown evenly with a pipette.
Bradford protein quantification
(1) The standard curve was constructed, and (2) 20 µl of protein solution was prepared: 1 µl of the tested sample was mixed with 19 µl of water, and each sample was tested for 2 replicates. (3) Then, 200 µl of the Bradford working solution was added to each replicate and mixed evenly. (4) At room temperature in the dark, the replicates stood for 15 min. The purple gradient was observed and detected at the 595-nm wavelength by a microplate reader. (5) The total amount of protein in the tested sample was calculated from the standard curve.
SDS-PAGE electrophoresis
To start, (1) 20 µg of the protein sample 5 : 1 (v/v) was mixed with 5× loading buffer, bathed in boiling water for 5 min, and then centrifuged at 14,000 rpm for 10 min. The supernatant was taken to conduct 10% SDS-PAGE electrophoresis. (2) Electrophoresis conditions: constant flow of 14 mA, electrophoresis duration of 90 min. (3) Comas brilliant blue staining.
Trypsin proteolysis
(1) A total of 60 µg of the protein solution was taken and placed in a centrifuge tube with 5 µl of 1 M DTT solution. The solution was shaken, mixed, and kept at 37°C for 1 h. Then, 20 µl of 1 M IAA solution was added, shaken, and mixed. The tube stood for 1 h in the dark at room temperature. (2) All samples to be tested were added to the ultra-filtration tube and centrifuged, and the collected solution was discarded. (3) Then, 100 µl of UA (pH 8.0, 8 M urea, 100 mM Tris-HCl) was added to the ultra-filtration tube and centrifuged. The collected solution was discarded and repeated twice, after which (4) 100 µl of 50 mM NH4HCO3 was added and centrifuged. The collected solution was discarded and repeated 3 times. (5) After changing the collection tube, trypsin was added to the ultra-filtration tube. The amount of trypsin was determined at the ratio of 50 : 1 between the protein and enzyme, and the solution was hydrolysed at 37°C for 12–16 h.
Liquid chromatography–mass spectrometry
A nanolitre-flow-rate, high-performance liquid chromatography (HPLC) system was adopted to separate the samples to be analysed. The chromatography column was balanced with 95% liquid A. Each sample was loaded into the pre-column of an Orbitrap Fusion Lumos mass spectrometer (database: uniprot-human_170221, Software: Proteome Discover) by the auto-sampler and separated by the analytical column. The samples were then separated by capillary HPLC and analysed.
Results
Results of protein quantification
The protein concentration, total protein amount, and electrophoretic amount in the CPPE group were all higher than those in the UPPE group, and the changes in their values were proportional to the OD absorbance values (Table I).
Results of SDS-PAGE electrophoresis
Compared with the UPPE group, the CPPE group had clearer bands around 10, 25, 60, 90, 100, 120, and 220 kDa after the high-abundance proteins were extracted, which was consistent with the expected results (Figure 1).
Figure 1
Results of protein electrophoresis. Note: A and B were the raw samples. A-1 and B-1 were the samples from which high-abundance proteins were extracted, by which the bands of high-abundance proteins were effectively removed. The total proteins in the 2 samples were effectively separated within the molecular weight range of 15–220 kDa, with no degradation of the proteins
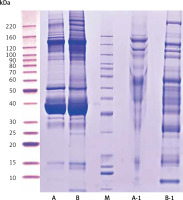
Results of liquid chromatography–mass spectrometry
The differential PPE proteins were investigated (Figure 2) and statistically analysed (Figures 3 and 4) after being identified in the 2 uploading repetitions. The differential proteins were determined under the condition of 1.5 difference multiples. An FC value ≥ 1.5 and p-value < 0.05 signified upregulation; FC ≤ 0.67 and p < 0.05 signified downregulation; and 0.67 < FC < 1.5 or p > 0.05 indicated no significant change in the expression level. The proteins with upregulated expression are illustrated in Table II, and the proteins with downregulated expression are shown in Table III.
Table II
Proteins with upregulated expressions
Table III
Proteins with downregulated expressions
Gene ontology (GO) analysis
A GO analysis is a series of classification systems based on gene function and is an important tool in the field of bioinformatics. This method has a complete set of control characters, the main purpose being to explain the key functions that eukaryotic genes and proteins perform in cells.
Gene ontology can be divided into 3 ontologies: (1) Cell components – A cell component is a structural object, mainly used to describe the sub-cellular structures (e.g. the nucleus) or protein production components (proteasome, ribosome, etc.). (2) Molecular functions – These are mainly used to describe the biological activities, such as catalytic reactions, that occur in cells. They can also represent the operations performed by specific objects without designating specific conditions such as when and where the operations occur. (3) Biological processes – These are events wherein many molecular functions are combined and coordinated. It may be difficult to strictly distinguish between biological processes and molecular functions, but, in general, biological processes require multiple different steps.
In the present study, a GO analysis was conducted on the identified proteins. The results showed that the upregulated differential proteins were mostly involved in the biosynthesis of monosaccharides, glucose catabolism, glycolysis of fructose-6-phosphate, glycolysis of glucose-6-phosphate, and NADH regeneration (Figure 5), while the downregulated differential proteins mostly participated in the processes of fibrinogen complexes, protein polymerization, and coagulation (Figure 6).
KEGG analysis
Organisms are composed of different kinds of proteins that perform their functions in a coordinated and orderly manner. The purpose of a pathway analysis is to better investigate the roles of proteins. One of the public databases about pathways is KEGG. Through pathway analysis, the functions of proteins in the process of biological activities are determined with the main participation in the metabolic pathways and signal transductions.
One method of pathway significant enrichment analysis is to use KEGG Pathway as the research unit and apply hyper-geometric testing to determine the pathways significantly enriched by differential proteins. Using this research method, the most important signal transduction and biochemical metabolic pathways involved with differential proteins can be determined. Herein, the KEGG function analysis was adopted to investigate the most prominent biochemical metabolic pathways and related signal transductions of the differential proteins in UPPE and CPPE. The results showed that CPPE had increased protein expressions in the HIF-1 signalling pathway and glycolysis/gluconeogenesis (Figure 7) but decreased expressions in platelet activation and complement activation (Figure 8).
Discussion
Human genome sequencing has been completed, and we have used genomics technology to conduct a large number of related studies on genes and diseases, bringing about more opportunities for the development of modern medicine. Proteomics is an emerging research field in the post-gene era. It is well known that although proteins are encoded by genes, they are affected by the internal and external environments and types of cells and are constantly changing. Moreover, due to RNA splicing, editing, or post-transcriptional modification, a single gene may be related to multiple proteins. Therefore, the relationship between genes and proteins is not completely linear; rather, they are 2 fields that complement each other and are closely correlated.
Thus far, the research fields of proteomics have mainly focused on the following aspects: (1) It is proteins, not genes, that determine the functions of cells. Proteomics can investigate the micro-features of proteins. While certain body fluids and plasma may not express much DNA or RNA, proteins may be present in large quantities, so proteomics may have advantages over genomics. (2) Protein isoforms and post-transcriptional modifications cannot be identified through genomic studies, but important protein information can be obtained through proteomic studies. Thus, changes in protein composition can be compared between pathological and normal conditions to find markers for the diagnosis of disease. (3) Regarding the study of protein–protein interactions, with the invention of biomass spectrometry, advances in medical informatics, and the establishment of relevant databases, we are now able to complete high-throughput proteomics studies [7, 8]. In recent years, rapid advances in molecular biology and bioinformatics have made it possible to identify and quantify any kind of trace protein, thus enabling efficient analysis of low-abundance proteins and post-translational modifications [9, 10]. Malignant tumours comprise the most extensive field of proteomics research. Researchers have begun to apply this technology to investigate tumour markers and have successfully discovered new protein markers for the diagnosis of malignant tumours [11]. In a previous study, leaky pleural effusions were used instead of normal pleural effusions as a control to study the expression of proteins expressed in malignant pleural effusions, and 7 differential proteins were found [12]. In respiratory diseases, proteomics is also the most widely used research method concerning malignant lung cancer, and several differentially expressed proteins have been found [13–16]. Currently, the research on CPPE is mostly focused on inflammatory cytokines such as tumour necrosis factor-α, IL-8, and IL-1 as well as neutrophil elastase, myeloperoxidase, metalloproteinase, and other enzymes [17–22], and there are a few studies on proteomics concerning pleural effusion. At present, the development of high-throughput proteomic technology provides technical support for the proteomic analysis of body fluids, which may help in the discovery of new biomarkers [23–25]. Moreover, emerging computer and bioinformatics technologies can analyse and interpret a large number of experimental proteomic data. These technological advances make it possible to investigate the proteomics of pleural effusion.
There are several high-abundance proteins in exudative pleural effusion, such as albumin, immunoglobulin, and α-antitrypsin. The presence of these high-abundance proteins may affect the expression of low-abundance proteins; thus, the presence of the former is not conducive to the detection of the latter, especially with the obvious interference of albumin [12]. Therefore, to ensure the reliability of an experiment, it is necessary to extract the high-abundance proteins [26]. However, this process also has a certain degree of negative impact because a small number of low-abundance proteins are combined with the high-abundance proteins, causing the loss of both protein types.
The application of proteomic technology in the field of PPE pathogenesis is still in the exploratory stage. The accumulated experience is not yet rich, and many research areas need to be explored. Currently, several researchers have begun to apply proteomic technology in the research of interstitial pulmonary diseases and pulmonary infectious diseases, and related research on proteomics has also begun in the study of ARDS [27, 28]. Therefore, we hope that through the study of proteomics, we will gain better understanding of the protein expression pattern, protein biological activity, metabolic pattern, and transmission pathway of PPE to understand the disease in depth. At present, the proteomics of pleural effusion has not been studied in depth, but more and more scholars have paid attention to it. Chiu et al. found that the level of glycolytic glucose in pleural effusion of patients with complex pleural effusion was significantly reduced, which was similar to the results of our study, and they also found that glucose and 3-hydroxybutyric acid could be used as markers to distinguish different metabolic pathways [29]. Based on proteomic analysis of pleural effusion near pneumonia, Hsueh found that dipeptides were closely related to the expression of related inflammatory factors in the pleural cavity, suggesting that dipeptides may be involved in the inflammatory response in the pleural cavity through immune regulation and affect the course of the disease [30]. By proteomic method, Chiu et al. found that in patients with pleural effusion near pneumonia, anaerobic glycolysis metabolism was enhanced, lactic acid and butyric acid were increased, and the increased expression of hypoxanthine may be related to the formation of fibrin in the pleural cavity [31]. In addition, the pleural effusion around simple pneumonia and complex pneumonia can be distinguished by protein spectrum analysis, and the people who need active treatment or even surgical treatment can be predicted [32, 33]. Through proteomics, Wu et al. found that the four markers of BPI, NGAL, AZU1, and calcium-guard protein are most significantly increased, among which BPI has the highest value in diagnosing CPPE, if combining with BPI and LDH, then the sensitivity and specificity to the diagnosis of CPPE will be increased to 100% and 91.4%, so the study of thoracic fluid protein histology of pneumonia may find more novel biomarkers [5].
The expressions of differential proteins were investigated in the present study. To increase the study’s representativeness, the pleural effusion samples of 10 patients in the study group and 10 in the control group were combined to form group A and group B, respectively. A similar method has been reported in related studies. Using label-free proteomic technology and bioinformatics analysis, it was determined that there were 257 proteins in total from the 2 groups, including 200 specific to the CPPE group, 21 specific to the UPPE group, and 38 upregulated and 29 downregulated in the CPPE group. A GO analysis showed that the upregulated differential proteins participated in the biosynthesis of monosaccharides, glucose catabolism, glycolysis of fructose-6-phosphate, glycolysis of glucose-6-phosphate, and NADH regeneration. The downregulated differential proteins were concentrated in the processes of fibrinogen complexes, protein polymerization, and coagulation. These results suggest that the glucose metabolism in the chest cavity of the CPPE patients was active and that NADH was also produced in glycolysis and the citric acid cycle in cell respiration. It is an important coenzyme for electron transfer in the body as well as an important part of the energy production chain in mitochondria control markers. Increased levels of the two often indicate metabolic imbalance. The downregulated differential proteins mostly participated in the processes of fibrinogen complexes, protein polymerization, and blood coagulation, suggesting that the coagulation process in the pleural cavity was enhanced and the formation of fibrin increased, which might be correlated with the infection and inflammation in the cavity. It was revealed in the KEGG analysis that the CPPE group had increased protein expressions in amino acid synthesis, the HIF-1 signalling pathway, and glycolysis/gluconeogenesis as well as weakened protein expressions in platelet activation and complement activation. These results were consistent with the results of the GO analysis. We considered that it might be correlated with intrapleural infection, enhanced bacterial metabolism, hypoxic stimulation, and imbalances in the blood coagulation system and immune system. Overall, the results suggested that proteomics could be used to investigate the pathogenesis of PPE and that new biomarkers that can distinguish between UPPE and CPPE might be discovered through subsequent verification experiments.
This study also has some limitations, such as a small sample size and insufficient representativeness. And into the group of patients with standard need to be further refinement, such as pneumonia side pleural effusion patients of different ages there may be some difference between protein content [33], so in a follow-up study, we need to further increase the sample size, at the same time reduce the influence of some confounding factors, and look forward to the future we can have better results.
In conclusion, proteomics is a new technology of the post-gene era, with high sensitivity and accuracy. However, it is still in its infancy in the research of pulmonary infectious diseases, and related research is not yet mature. In this study, 38 upregulated proteins and 29 downregulated proteins were found in the pleural effusions of patients with PPE. Moreover, it was found that glucose and amino acid metabolism, NADH regeneration, and the HIF-1 signalling pathway in the effusions of patients with CPPE were enhanced, while protein polymerization, blood coagulation, platelet activation, and complement activation were weakened. These results suggest that there were obvious signs of infection in the pleural cavity in the CPPE group. The process of infection was accompanied by changes such as hypoxia and abnormal energy metabolism in addition to obvious inflammation and inflammatory disorders in the pleural cavity.